Can AI be developed and used responsibly? New case studies put policy into practice
Almost exactly two years ago, OpenAI launched ChatGPT, and the world hurtled into a new era. Virtually every sector is finding groundbreaking uses for AI. Tech behemoths are vying for dominance as a surge of AI startups with skyrocketing valuations jockey for position. Generative AI is set to become a $1.3 trillion market by 2032, up from $40 billion in 2022. The technology’s capabilities continue to advance at an astonishing pace — just compare 2023’s viral AI-generated video of Will Smith eating spaghetti to 2024 demos by Sora and Runway.
This breakneck speed of growth and innovation has created an urgent need for policy guardrails to mitigate consequences as varied as mass surveillance, disinformation, and job displacement. But governments worldwide are scrambling to keep up. “Regulating AI, and even more specifically synthetic media, is a bit like saying you want to regulate water, or air, since AI is such a big, impactful technology,” said Claire Leibowicz, Head of AI and Media Integrity at the Partnership on AI (PAI), a global multi-stakeholder nonprofit building best practices for the responsible development and use of AI.
To take on some of AI’s grand challenges, PAI is casting an enormous net. With more than 120 institutions (including Adobe) across academic, civil society, industry, and media organizations, the partnership aims to create guidance and solutions informed by diverse perspectives and collective wisdom. The overarching principle is that AI can and should contribute to a more just, equitable, and prosperous world.
On November 19, PAI released a new set of case studies that illustrate the practical application of its guidelines in various sectors, with participation from Meta, Microsoft, Truepic, Thorn, and researchers from the Stanford Institute for Human-Centered AI. The case studies outline various considerations around disclosing content and navigating harmful uses of synthetic media, from authenticating cultural heritage imagery in conflict zones to mitigating the risk of generative AI models creating child sexual abuse material.
The Content Authenticity Initiative (CAI) and PAI work in complementary spaces, and both organizations aim to unite many disparate entities in pursuit of a greater good. I spoke with Leibowicz to learn more about PAI’s approach to this massive endeavor, and how to get started in AI policy.
“I grew up thinking that different ideas matter. Disagreement should be welcome, but we ultimately need to channel that into something that serves society,” she said. “Figuring out how to do that animates me.”
This interview has been edited for length and clarity.
Tell me a little about your background. How did you end up in the field of policy and AI?
I credit my high school teachers with fueling my interest in behavioral science research early on. I did research on trustworthiness judgments — specifically which features we use to determine who is trustworthy, like facial expressions or characteristics of speech. That’s when I first started thinking about how technology was changing how we make social judgments, without many of these cues present when interacting online.
In college, I started doing a lot of cognitive science research about how groups form, and how intergroup empathy and antipathy take shape in the brain. Technology was touching everything about how people interact: how they work together, decide to date, determine who to trust on the news, etc.
Ultimately, I found my way into computer science classrooms where I encountered AI; I loved how it was both a metaphor for thinking about human cognition, and something that would affect human interaction itself. Working in AI policy, I realized, would allow me to think about the big-picture behavioral challenges I’ve always been interested in, while having potential impact on fields as varied as education, the arts, democracy, and economics.
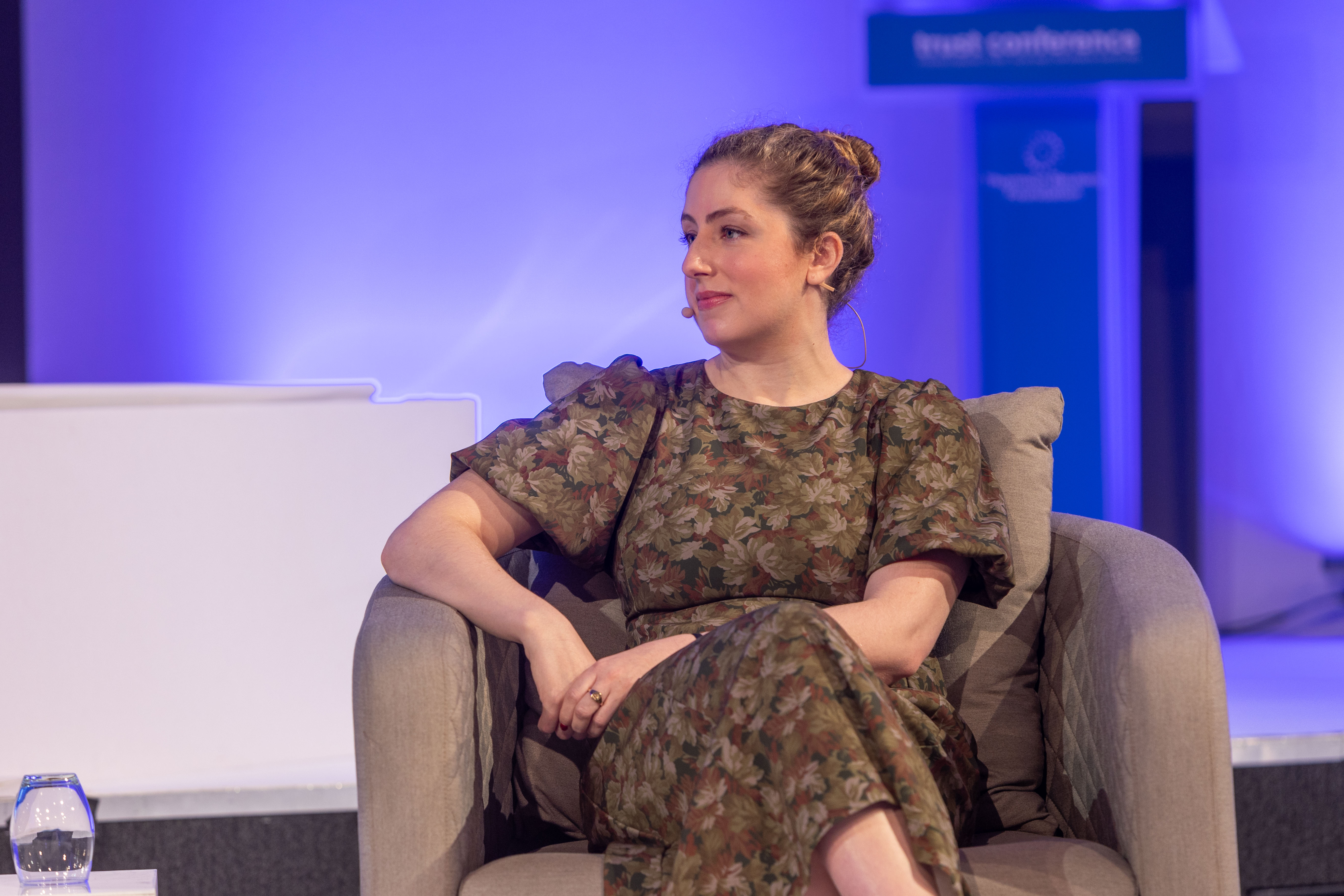
Claire Leibowicz, Head of AI and Media Integrity at the Partnership on AI, speaks at the Thomson Reuters Trust Conference in London. Photo credit: Copland-Cale Photography
You turned policy commitments to action among the biggest technology companies. Tell us about PAI’s Synthetic Media Framework and the process involved in developing it.
The Framework comes from four years of work bringing together over 100 different viewpoints on generative media’s impact on many fields — news, public safety, and intimate image abuse, for example. We figured out a collective set of values that could be translated into practices for how to responsibly build synthetic media tools, create content, and distribute it.
We worked with this multi-stakeholder cohort to delineate guidance on content transparency, consent, a set of responsible and harmful use cases around synthetic media, and labeling protocols for helping audiences understand content. Meaningfully, we required the 18 signatories of that effort — which ranged from Bumble, Adobe, the BBC, and civil society organizations — to contribute a case study about how they enacted that guidance.
How did you go about selecting these different institutions? To what extent were policymakers involved?
Policymakers were not actively involved in the development of the Framework, but they were an intended audience. Some of that has to do with the timing. We began this work in 2019, well before ChatGPT launched, when there wasn’t as much policy energy devoted to this topic.
Importantly, this voluntary Framework doesn’t replace regulation or policymaker activity, but we wanted to get ahead of that work and show what respective actors were doing. We wanted a balance of builders, creators, and distributors. We wanted a group that was illustrative of the very different types of institutions that play a role — sometimes an outsize role — in how people encounter synthetic media. For example, showing how a newsroom might be affected by what an AI model builder does far upstream.
PAI’s previous set of case studies putting the Framework into practice, which was released in March 2024, focused on transparency, consent, and harmful/responsible uses of synthetic media. How does this newest set differ?
These new cases focus on direct disclosure, or in other words, how we convey to people that content has been generated or manipulated with AI — for example, using Content Credentials. We realized that this has been underexplored in the realm of synthetic media governance. Even if we had perfect technical signals for showing how content moved across the web, we would need to then convey that to human beings, and a lot of formal policies aren’t clarifying how to do that in a meaningful way.
"One theme that emerged is that people don’t necessarily just want to know if content has been AI-generated. They want to know if it’s been edited in a way that matters to them, changes the meaning of content, or is deceptive."
Claire Leibowicz
What are some of the main themes that have emerged?
One theme that emerged is that people don’t necessarily just want to know if content has been AI-generated. They want to know if it’s been edited in a way that matters to them, changes the meaning of content, or is deceptive. A lot of people don’t care to know about basic color correction or cosmetic changes — what they care about is materiality and deception. Obviously, it’s really hard to define that, but some of the cases show that certain categories like elections or current events might require different, or more salient, labeling.
A second theme is that we need descriptive context about content, whether AI-generated or not. We saw in Meta’s case that they were labeling things as “made with AI,” but they received a lot of backlash from creators who were making minor changes that didn’t warrant that label. People really want to know the difference between “AI-edited” and “AI-generated.”
Ultimately, there is a need for user education. There needs to be broader coordination, broader emphasis from civic institutions, and better sharing of user research on how people respond to these labels – while remaining clearheaded about the limits of labels overall.
Taking into account that different user groups have different needs, are there any situations in which more descriptive context for direct disclosure would not help?
Thorn and Stanford HAI focused on how direct disclosure doesn’t help that much for certain categories of media like child sexual abuse material. It may help trust and safety officials or law enforcement prioritize what to investigate, but at the end of the day, a bad actor isn’t going to label that content. That’s why upstream harm mitigation is important as we’re thinking about direct disclosure.
What have been some notable impacts the Framework has had in influencing synthetic media development, creation, and distribution practices? How has the Framework complemented other standards and policy efforts?
The Framework has been referenced in policy and practice development by Meta, Google, OpenAI, and many other builders and distributors of synthetic media technology. Newsrooms have turned to our Framework to develop their own AI principles and practices, highlighted clearly with the BBC and the CBC in their case reports.
In government policy, we’re involved in the US AI Safety Institute at the National Institute of Standards and Technology (NIST), and have greatly shaped the thinking and writing of the Synthetic Content Working Group. Other government agencies in the US like the Federal Trade Commission along with legislators have incorporated our guidance on synthetic media, as have those in the UK and Europe, and multinational bodies like the UN and OECD.
How has PAI supported the CAI’s mission?
We’ve helped to catalyze adoption of the C2PA standard and other content authenticity efforts through the culture change, and sociotechnical exploration, that has emerged from this work. Having that precedent from a neutral third party to talk about these issues was really important to driving media transparency forward. For instance, we followed up on the Framework with candid conversations — very technical in nature while still grounded in social impact — about the different methods for adding provenance information to content, like watermarking and metadata. This helped the field with a shared vocabulary that has been used by practitioners, journalists, and policymakers. Perhaps most importantly, it built trust amongst these communities.
As AI ethics is such a quickly evolving field, what kind of adaptations do you think could be necessary to the Framework over time?
Lots of questions are emerging about how we will transpose some of these ideas about content transparency onto more interactive types of media beyond still images and videos. What will it be like when there is more personalization through chatbots or agentic AI? How do you balance the need to prove personhood or identity with safeguarding privacy?
Other considerations have to do with this big, open question about what people will respond to about AI-edited artifacts in media. How do we help people understand that just because something was edited with AI, it’s not necessarily untrue? Then there are many other domains of impact that haven't been explored in the Framework yet: legal infrastructure and financial services, to name a couple. And on the input side, how the models undergirding synthetic media will be trained and what data they can leverage from publishers and creatives.
What advice would you give to those interested in being involved in AI ethics or policy?
Having technical literacy has been empowering and helpful for me. At the same time, I’m a big believer in not thinking of AI ethics as a distinctive field, or a technical one, but one that is a conduit for many other fields of interest.
Stay curious about other facets of society. I’ve been surprised by how my interests in other fields — like art and history — shed light on my day job. And lastly, be ready for things to change all the time. Be open to learning, always feeling like you’re one step behind, and maybe getting comfortable in that feeling. And have a sense of humor. I’ve found that helps with everything!