July 2024 | This Month in Generative AI: Moving Through the Uncanny Valley (Pt. 2 of 2)
News and trends shaping our understanding of generative AI technology and its applications.
Last month I discussed how AI-generated images are passing through the uncanny valley. This month I'll discuss AI-generated voices and where they are in their journey from the creepy, robot-like voices of a few years ago to today’s more realistic outputs.
A prototypical text-to-speech system consists of two basic parts. First, a text is specified and typically converted into a phonetic and prosodic representation that captures the specific sounds, intonation, stress, and rhythm to be spoken. Second, a synthesis engine converts this symbolic representation into a raw audio waveform, typically through an intermediate frequency-based representation.
Synthesized voices have come a long way. Boosted by advances in machine learning, today's synthetic voices are increasingly more realistic. Perhaps most impressive is that voices can be cloned even if they weren't used during the training of the generative-AI system, and with as little as 30 seconds of a voice recording.
In collaboration with Sarah Barrington, a Ph.D. student at UC Berkeley, we have launched a new study to determine just how realistic these AI-generated voices are and whether a cloned voice sounds like the original speaker's voice.
In this study, participants listen to a set of voices (one at a time), half of which are real and half of which are AI-generated. Although we are still collecting data, we have completed a pilot study with 50 participants who each listened to a total of 40 short voice recordings. The average accuracy on this task was 65%, slightly better than chance performance of 50%. There was only a small bias in which accuracy for real voices was 68% and accuracy for fake voices was 62%. In other words, participants were slightly more likely to say a recording was real. You can test yourself on a set of 16 voices to see how you do.
We also asked participants how they thought they were distinguishing the real from the fake. We received some interesting insights, including:
- The person was breathing or taking breaths between words.
- Fake had too much enunciation.
- Fake had no speaking errors.
While these preliminary results suggest that AI-generated voices are passing through the uncanny valley, they do not mean that all AI-generated voices are indistinguishable from reality. The snippets of voices that participants heard were relatively short, between 3 and 10 seconds, and did not feature yelling, laughing, or anything that reflected strong emotions. If, however, generative AI continues along its current trajectory, it seems likely that sooner or later it is going to be very difficult to perceptually distinguish the real from the fake.
At the same time, AI-generated videos are still on the other side of the uncanny valley. For example, Runway ML recently dropped Gen-3 Alpha, their latest text-to-video generation model. Although at first glance the videos are pretty impressive and some of the short-term temporal consistency problems have been eliminated, there are problems with longer-term temporal consistency. For example, over a 10-second clip, the body shape of the man in pink changes dramatically (and sunglasses magically appear), and on the right the woman's race changes in the span of a few seconds midway through the video.
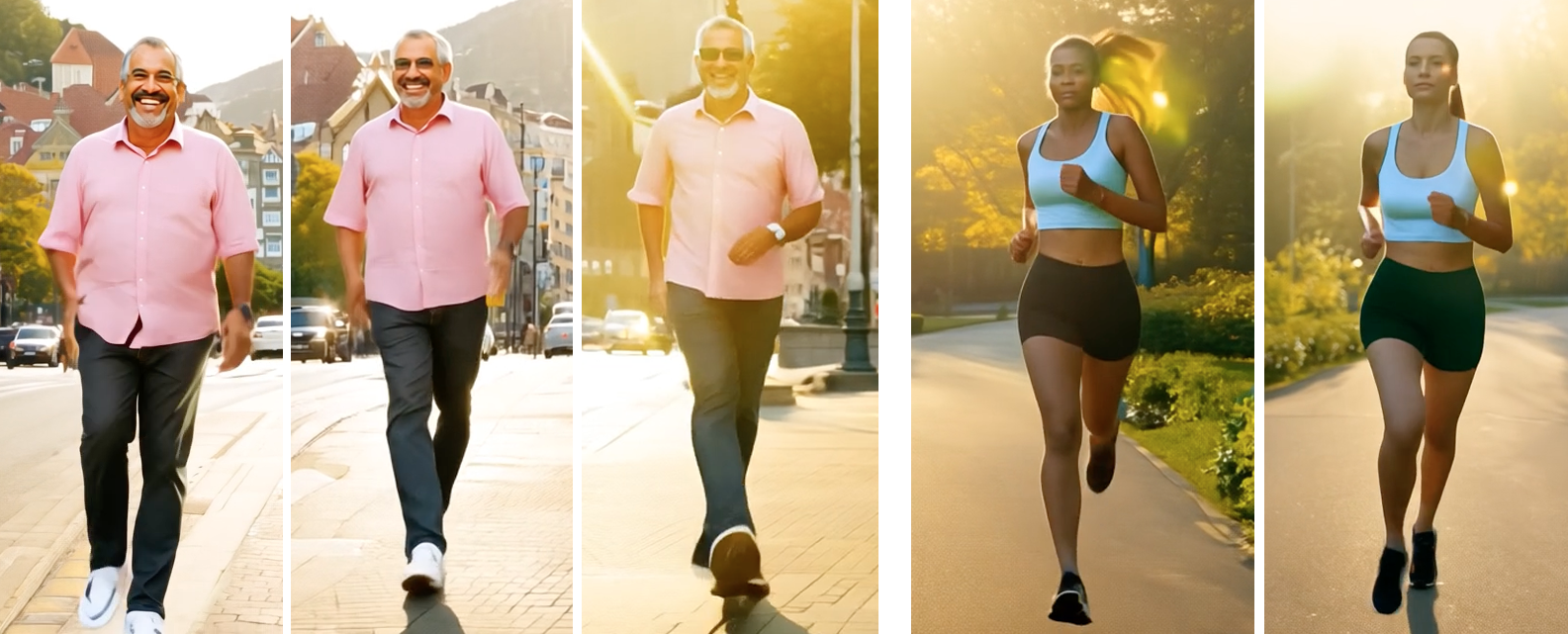
As with AI-generated images, creators can add Content Credentials to AI-generated audio files to make them easier to identify. One popular voice cloning service, Respeecher, has already implemented Content Credentials to help mitigate the weaponization of AI-generated voices. Other popular services like ElevenLabs offer classifiers that can determine whether a recording was created by their generative engines. And, of course, we and the broader digital forensic research community continue to develop the next generation of forensic tools for automatically detecting AI-generated voices.
The combination of credential-based and forensic-based solutions promises to mitigate the threats posed by generative AI. But since these solutions can’t eliminate the threats, consumer awareness and vigilance remain critical.
Author bio: Professor Hany Farid is a world-renowned expert in the field of misinformation, disinformation, and digital forensics. He joined the Content Authenticity Initiative (CAI) as an advisor in June 2023. The CAI is an Adobe-led community of media and tech companies, NGOs, academics, and others working to promote adoption of the open industry standard for content authenticity and provenance.
Professor Farid teaches at the University of California, Berkeley, with a joint appointment in electrical engineering and computer sciences at the School of Information. He’s also a member of the Berkeley Artificial Intelligence Lab, Berkeley Institute for Data Science, Center for Innovation in Vision and Optics, Development Engineering Program, and Vision Science Program, and he’s a senior faculty advisor for the Center for Long-Term Cybersecurity. His research focuses on digital forensics, forensic science, misinformation, image analysis, and human perception.
He received his undergraduate degree in computer science and applied mathematics from the University of Rochester in 1989, his M.S. in computer science from SUNY Albany, and his Ph.D. in computer science from the University of Pennsylvania in 1997. Following a two-year post-doctoral fellowship in brain and cognitive sciences at MIT, he joined the faculty at Dartmouth College in 1999 where he remained until 2019.
Professor Farid is the recipient of an Alfred P. Sloan Fellowship and a John Simon Guggenheim Fellowship, and he’s a fellow of the National Academy of Inventors.