March 2024 | This Month in Generative AI: Text-to-Movie
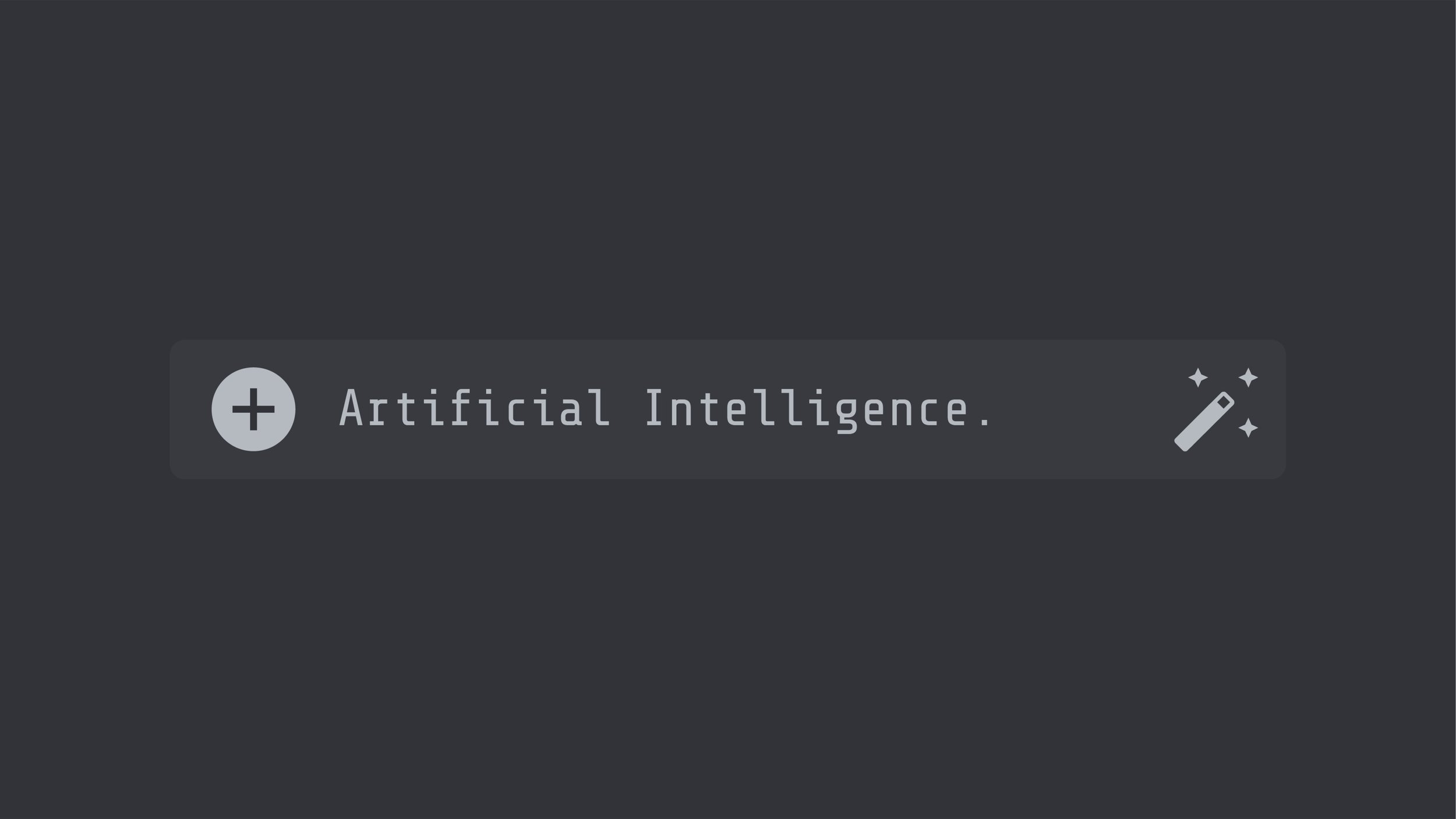
News and trends shaping our understanding of generative AI technology and its applications.
Generative AI embodies a class of techniques for creating audio, image, or video content that mimics the human content creation process. Starting in 2018 and continuing through today, techniques to generate highly realistic content have continued their impressive trajectory. In this post, I will discuss some recent breakthroughs in a category of techniques that generate images, audio, and video from a simple text prompt.
Faces
A common computational technique for synthesizing images involves the use of a generative adversarial network (GAN). StyleGAN is, for example, one of the earliest successful systems for generating realistic human faces. When tasked with generating a face, the generator starts by laying down a random array of pixels and feeding this first guess to the discriminator. If the discriminator, equipped with a large database of real faces, can distinguish the generated image from the real faces, the discriminator provides this feedback to the generator. The generator then updates its initial guess and feeds this update to the discriminator in a second round. This process continues with the generator and discriminator competing in an adversarial game until an equilibrium is reached when the generator produces an image that the discriminator cannot distinguish from real faces.
Below are representative examples of GAN-generated faces. In two earlier posts, I discussed how photorealistic these faces are and some techniques for distinguishing real from GAN-generated faces.
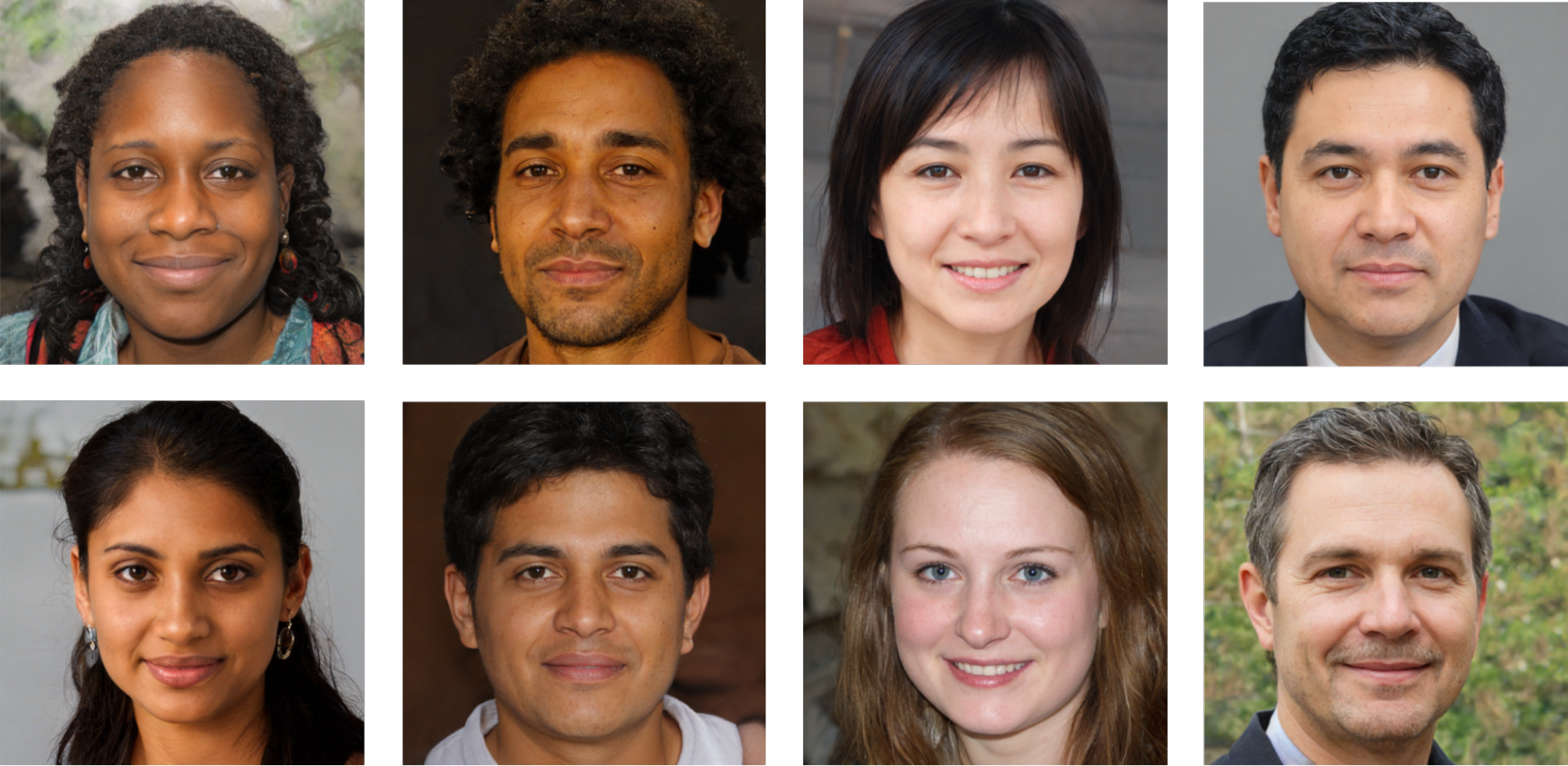
Text-to-image
Although they produce highly realistic results, GANs do not afford much control over the appearance or surroundings of the synthesized face. By comparison, text-to-image (or diffusion-based) synthesis affords more rendering control. Models are trained on billions of images that are accompanied by descriptive captions, and each training image is progressively corrupted until only visual noise remains. The model then learns to denoise each image by reversing this corruption. This model can then be conditioned to generate an image that is semantically consistent with a text prompt like “Pope Francis in a white Balenciaga coat.”
From Adobe Firefly to OpenAI's DALL-E, Midjourney to Stable Diffusion, text-to-image generation is capable of generating highly photorealistic images with increasingly fewer obvious visual artifacts (like hands with too many or too few fingers).
Text-to-audio
In 2019, researchers were able to clone the voice of Joe Rogan from eight hours of voice recordings. Today, from only one minute of audio, anyone can clone any voice. What is most striking about this advance is that unlike the Rogan example, in which a model was trained to generate only Rogan's voice, today's zero-shot, multi-speaker text-to-speech can clone a voice not seen during training. Also striking is the easy access to these voice-cloning technologies through low-cost commercial or free open-source services. Once a voice is cloned, text-to-audio systems can convert any text input into a highly compelling audio clip that is difficult to distinguish from an authentic audio clip. Such fake clips are being used for everything from scams and fraud to election interference.
Text-to-video
A year ago, text-to-video systems tasked with creating short video clips from a text prompt like "Pope Francis walking in Times Square wearing a white Balanciaga coat" or "Will Smith eating spaghetti'' yielded videos of which nightmares are made. A typical video consists of 24 to 30 still images per second. Generating many realistic still images, however, is not enough to create a coherent video. These earlier systems struggled to create temporally coherent and physically plausible videos in which the inter-frame motion was convincing.
However, just this month researchers from Google and OpenAI released a sneak peek into their latest efforts. While not perfect, the resulting videos are stunning in their realism and temporal consistency. One of the major breakthroughs in this work is the ability to generalize existing text-conditional image models to train on entire video sequences in which the characteristics of a full space-time video sequence can be learned.
In the same way that text-to-image models extend the range of what is possible as compared to GANs, these text-to-video models extend the ability to create realistic videos beyond existing lip-sync and face-swap models that are designed specifically to manipulate a video of a person talking.
Text-to-audio-to-video
Researchers from the Alibaba Group released an impressive new tool for generating a video of a person talking or singing. Unlike earlier lip-sync models, this technique requires only a single image as input, and the image is then fully animated to be consistent with any audio track. The results are remarkable, including a video of Mona Lisa reading a Shakespearean sonnet.
When paired with text-to-audio, this technology can generate, from a single image, a video of a person saying (or singing) anything the creator wishes.
Looking ahead
I've come to learn not to make bold predictions about when and what will come next in the space of generative AI. I am, however, comfortable predicting that full-blown text-to-movie (combined audio and video) will soon be here, allowing for the generation of video clips from text such as: "A video of a couple walking down a busy New York City street with background traffic sounds as they sing Frank Sinatra's New York, New York." While there is much to be excited about on the content creation and creativity side, legitimate concerns persist and need to be addressed.
While there are clear and compelling positive use cases of generative AI, we are already seeing troubling examples in the form of people creating non-consensual sexual imagery, scams and frauds, and disinformation.
Some generative AI systems have been accused of infringing on the rights of creators whose content has been ingested into large training data sets. As we move forward, we need to find an equitable way to compensate creators and to give them the ability to opt in to or out of being part of training future generative AI models.
Relatedly, last summer saw a historic strike in Hollywood by writers and performers. A particularly contentious issue centered around the use (or not) of AI and how workers would be protected. The writers’ settlement requires that AI-generated material cannot be used to undermine a writer’s credit, and its use must be disclosed to writers. Protections for performers include that studios give fair compensation to performers for the use of digital replicas, and for the labor unions and studios to meet twice a year to assess developments and implications of generative AI. This latter agreement is particularly important given the pace of progress in this space.
Author bio: Professor Hany Farid is a world-renowned expert in the field of misinformation, disinformation, and digital forensics. He joined the Content Authenticity Initiative (CAI) as an advisor in June 2023. The CAI is an Adobe-led community of media and tech companies, NGOs, academics, and others working to promote adoption of the open industry standard for content authenticity and provenance.
Professor Farid teaches at the University of California, Berkeley, with a joint appointment in electrical engineering and computer sciences at the School of Information. He’s also a member of the Berkeley Artificial Intelligence Lab, Berkeley Institute for Data Science, Center for Innovation in Vision and Optics, Development Engineering Program, and Vision Science Program, and he’s a senior faculty advisor for the Center for Long-Term Cybersecurity. His research focuses on digital forensics, forensic science, misinformation, image analysis, and human perception.
He received his undergraduate degree in computer science and applied mathematics from the University of Rochester in 1989, his M.S. in computer science from SUNY Albany, and his Ph.D. in computer science from the University of Pennsylvania in 1997. Following a two-year post-doctoral fellowship in brain and cognitive sciences at MIT, he joined the faculty at Dartmouth College in 1999 where he remained until 2019.
Professor Farid is the recipient of an Alfred P. Sloan Fellowship and a John Simon Guggenheim Fellowship, and he’s a fellow of the National Academy of Inventors.