From the darkroom to generative AI
In this era of generative AI, how do we determine whether an image is human-created or machine-made? For the Content Authenticity Initiative (CAI), Professor Hany Farid shares the techniques used for identifying real and synthetic images — including analyzing shadows, reflections, vanishing points, environmental lighting, and GAN-generated faces. A world-renowned expert in the field of misinformation, disinformation, and digital forensics as well as an advisor to the CAI, Professor Farid explores the limits of these techniques and their part in a larger ecosystem needed to regain trust in the visual record.
This is the first article in a six-part series.
More from this series:
Part 1: From the darkroom to generative AI
Part 2: How realistic are AI-generated faces?
Part 3: Photo forensics for AI-generated faces
Part 4: Photo forensics from lighting environments
Part 5: Photo forensics from lighting shadows and reflections
Part 6: Passive versus active photo forensics in the age of AI and social media
by Hany Farid
Stalin, Mao, Hitler, Mussolini, and many other dictators had photographs manipulated in an attempt to rewrite history. These men understood the power of photography: If they changed the visual record, they could potentially change history.
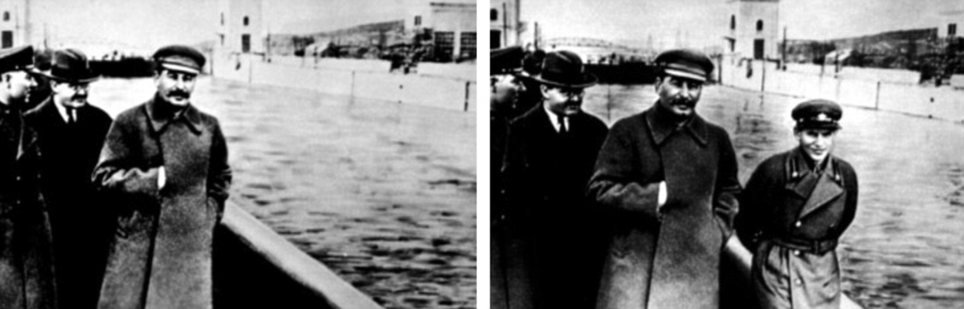
In the past, altering the historical record required cumbersome and time-consuming darkroom techniques. Starting in the early 2000s, powerful and low-cost digital technology began to make it easier to record and alter digital images. And today, sophisticated generative-AI software has fully democratized the ability to create compelling digital fakes.
Twenty-five years ago, I was waiting in line at the library when I noticed an enormous book in the return cart called The Federal Rules of Evidence. I thumbed through the book and came across Rule 1001 of Article X, which outlined the rules under which photographic evidence can be introduced in a court of law. The rules seemed straightforward until I read the definition of original:
An “original” of a writing or recording means the writing or recording itself or any counterpart intended to have the same effect by the person who executed or issued it. For electronically stored information, “original” means any printout — or other output readable by sight — if it accurately reflects the information.
I was struck that the definition of original included this vague statement: “... or other output readable by sight.”
At the time, digital cameras and digital editing software were still primitive by today’s standards, and generative AI was unimaginable. The trajectory of technology, however, was fairly clear, and it seemed to me that advances in the power and ubiquity of digital technology would eventually lead to complex issues around how we can trust digital media. As we enter the new age of generative AI, these issues have only become more salient.
Although it varies in form and creation, generative AI content (a.k.a. deepfakes) refers to images, audio, or video that has been automatically synthesized by an AI-based system. Deepfakes are the latest in a long line of techniques used to manipulate reality — from Stalin's darkroom to Photoshop to classic computer-generated renderings. However, their introduction poses new opportunities and risks now that everyone has access to what was historically the purview of a small number of sophisticated organizations.
Even in these early days of the AI revolution, we are seeing stunning advances in generative AI. The technology can create a realistic photo from a simple text prompt, clone a person's voice from a few minutes of an audio recording, and insert a person into a video to make them appear to be doing whatever the creator desires. We are also seeing real harms from this content in the form of non-consensual sexual imagery, small- to large-scale fraud, and disinformation campaigns.
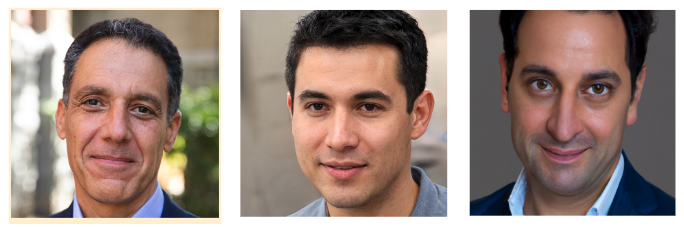
Building on our earlier research in digital media forensics techniques, over the past few years my research group and I have turned our attention to this new breed of digital fakery. All our authentication techniques work in the absence of digital watermarks or signatures. Instead, they model the path of light through the entire image-creation process and quantify physical, geometric, and statistical regularities in images that are disrupted by the creation of a fake.
In this series of posts, I will describe a collection of different techniques that we use to determine whether an image is real or fake. I will explain the underlying analyses — including analyzing shadows, reflections, vanishing points, specular reflections in the eye, and AI-generated faces — and the conditions under which the analyses are suitable.
While these and related techniques can be powerful, I need to emphasize their limitations.
First, it is important to understand that while the presence of evidence of manipulation can tell us something, the absence of traces of manipulation do not prove that an image is real. We cannot differentiate between a flawless fake and the real thing.
Second, nearly all forensic techniques have a limited shelf life because techniques to manipulate content are continually improving. So while these techniques are applicable today, we have to continually be aware of how generative AI is evolving.
Finally, these forensic techniques are only one part of a larger ecosystem needed to regain trust in the visual record. This includes efforts like the Content Authenticity Initiative (to which I contribute), some regulatory pressures, education, and the responsible deployment of AI technologies.
Further reading:
[1] H. Farid. Image Forensics. Annual Review of Vision Science, 5(1):549-573, 2019.
More from this series:
Part 2: How realistic are AI-generated faces?
Part 3: Photo forensics for AI-generated faces
Part 4: Photo forensics from lighting environments
Part 5: Photo forensics from lighting shadows and reflections
Part 6: Passive versus active photo forensics in the age of AI and social media
Author bio: Professor Hany Farid is a world-renowned expert in the field of misinformation, disinformation, and digital forensics. He joined the Content Authenticity Initiative (CAI) as an advisor in June 2023. The CAI is a community of media and tech companies, non-profits, academics, and others working to promote adoption of the open industry standard for content authenticity and provenance.
Professor Farid teaches at the University of California, Berkeley, with a joint appointment in electrical engineering and computer sciences at the School of Information. He’s also a member of the Berkeley Artificial Intelligence Lab, Berkeley Institute for Data Science, Center for Innovation in Vision and Optics, Development Engineering Program, and Vision Science Program, and he’s a senior faculty advisor for the Center for Long-Term Cybersecurity. His research focuses on digital forensics, forensic science, misinformation, image analysis, and human perception.
He received his undergraduate degree in computer science and applied mathematics from the University of Rochester in 1989, his M.S. in computer science from SUNY Albany, and his Ph.D. in computer science from the University of Pennsylvania in 1997. Following a two-year post-doctoral fellowship in brain and cognitive sciences at MIT, he joined the faculty at Dartmouth College in 1999 where he remained until 2019.
Professor Farid is the recipient of an Alfred P. Sloan Fellowship and a John Simon Guggenheim Fellowship, and he’s a fellow of the National Academy of Inventors.